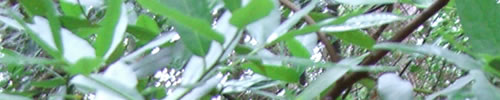
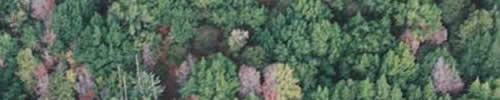

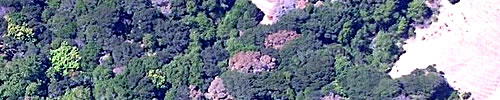
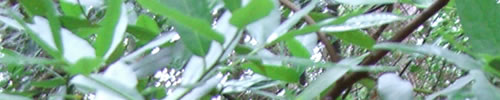
Sudden Oak Death Research
Sudden Oak Death is a new and virulent disease affecting hardwood forests in coastal California. The pathogen, Phytophthora ramorum, has reached epidemic levels in several California forests, killing thousands of trees. My lab has been a leader in using innovative combination of field data, remote sensing, spatial analysis, and internet technologies to understand patterning of disease through time, uncover critical ecological factors that lead to disease establishment and spread, and to deliver needed information on this important disease to stakeholders. My research in this area has broadened the understanding of SOD disease dynamics from sub-hectare to landscape scales, and has confirmed the importance of spatial dependence between mortality and other foliar hosts found in smaller scale field approaches.
My lab have published numerous papers on these topics (found here). Some of our earlier papers focused on the optimal sensor and resolution to map oak mortality. We first used digital imagery (ADAR 5500) (Spectral Bands: Blue: 450-550 nm, Green: 520-610 nm, Red: 610-700 nm, Near Infrared (NIR): 780-920 nm), at 1-m spatial resolution to map dead crowns, and forest structure, and also investigated hyperspectral imagery (CASI), and field spectrometry. We investigated different automated classifiers, including standard MLC and ISODATA, and comparing them to hybrid methods, object oriented methods, and machine learning classifiers. We also developed a novel tool for more accurately registering multiple airborne imagery scenes, which is a necessary first step before change detection can be performed.
forest stand with objects depicting dead crowns and bay trees. typical pixel sizes also shown.Once an adequate protocol for mapping crowns (and other disease hosts) was found (using object-based approaches: see figure), we could investigate patterns in mortality across space and time, and examine the spatial relationships between foliar hosts and terminal hosts over large scales. For example, we used multiple years’ imagery to map forest mortality using a novel spatial-temporal classification algorithm. In a third, we used the results from Dr. Guo’s novel object-based mapping work of oak mortality, and developed a method to track individual disease-related forest gaps through time. We have also been examining the potential niches for the disease over larger scales: the article with Qinghua Guo describes the first example of Support Vector Machines, a new Machine Learning tool, being used for predictive environmental niche modeling. We used this new method to map the potential spread of SOD in California, and nationwide. Desheng Liu and I also collaborated with Drs Wood and McPherson and investigated the spatial-temporal details that were collected by Dr McPherson in China Camp State Park, but had not been directly analyzed. In one paper we used univariate and multivariate spatial point pattern analyses to characterize and model the spatial dependence between dead oak trees, and between dead oak trees and California bay trees (an important host for the pathogen) through time. The results showed that new dead oak trees tend to be located within up to 300 m of past dead oak trees; and that a strong spatial association between oak tree mortality and California bay trees exists 150 m away. In another paper, we used two-dimensional spatial analysis tools to investigate the interaction across landscape scales of disease symptomology in coast live oaks and tree mortality through time, showing a link between early beetle infestation and later oak mortality.
We have also used the SOD program to develop innovated webGIS and digital sharing methods. We used the OakMapper site to refine a framework for participatory research that we present in a paper in Frontiers in Ecology and Environment and we focused the discussion on open-source tools in another paper published in Ecological Informatics.